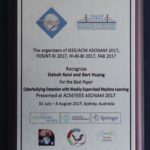
Elaheh Raisi, a computer science Ph.D. student in the Discovery Analytics Center and her advisor, Bert Huang, assistant professor in the Department of Computer Science, were recently honored with the Best Paper Award at the 2017 IEEE/Association for Computing Machinery International Conference on Advances in Social Networks Analysis and Mining (ASONAM), in Sydney, Australia.