Sanghani Center Student Spotlight: Haohui Wang
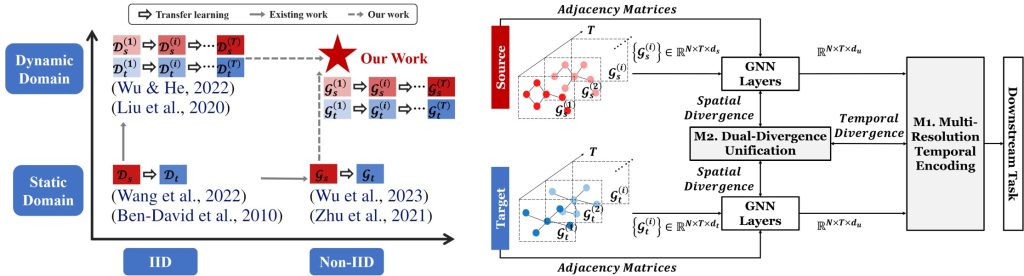
Attendees at two major conferences this summer will be hearing about Haohui Wang’s research.
Wang, a Ph.D. student advised by Dawei Zhou, will be traveling to Europe to present her collaborative work, “EvoluNet: Advancing Dynamic Non-IID Transfer Learning on Graphs,” at the 2024 International Conference on Machine Learning (ICML) in Vienna, Austria, in July; and ““Mastering Long-Tail Complexity on Graphs: Characterization, Learning, and Generalization” at the 2024 ACM SIGKDD International Conference on Knowledge Discovery and Data Mining in Barcelona, Spain, in August.
She will also present the ICML paper remotely at a Deloitte AI journal club, a group within Deloitte that discusses state-of-the-art AI techniques.
“As a Sanghani Center student, I have had the excellent opportunity of working with leading researchers in the field of artificial intelligence,” said Wang. “The center’s interdisciplinary environment fosters collaboration with experts across various related areas, enhancing the real-world application of my research.”
Her research focuses on advancing machine learning techniques, particularly in the areas of transfer learning and long-tail learning, proposing a framework to improve model performance on both head and tail classes. Both papers she is presenting at the summer conferences consider the challenges related to data, especially in real-world scenarios where labeled data is scarce.
“This makes our work applicable to various real-world scenarios, such as financial fraud detection. In financial transaction networks, while most transactions – such as credit card payments, and wire transfers – are normal, the rare occurrences of fraudulent transactions which include money laundering, synthetic identity transactions, are crucial to detect. We achieve improved detection by leveraging the models we have developed,” said Wang.
Her interest in this research area began during her undergraduate studies, particularly through coursework focused on finding the internal relationship and mechanism between daily things.
“I found it enjoyable to leverage the power of data science to solve real-world problems. This interest deepened during my master’s studies, leading me to focus my research efforts in machine learning ever since,” she said.
Wang earned a bachelor of science degree from Shandong University and a master of science degree from Zhejiang University, both in China.
Projected to graduate in May 2027, Wang said she will explore any opportunities in academia or industry that provide an opportunity to continue her research.