Sanghani Center graduate students gain real-world experience while working at companies and labs from coast to coast
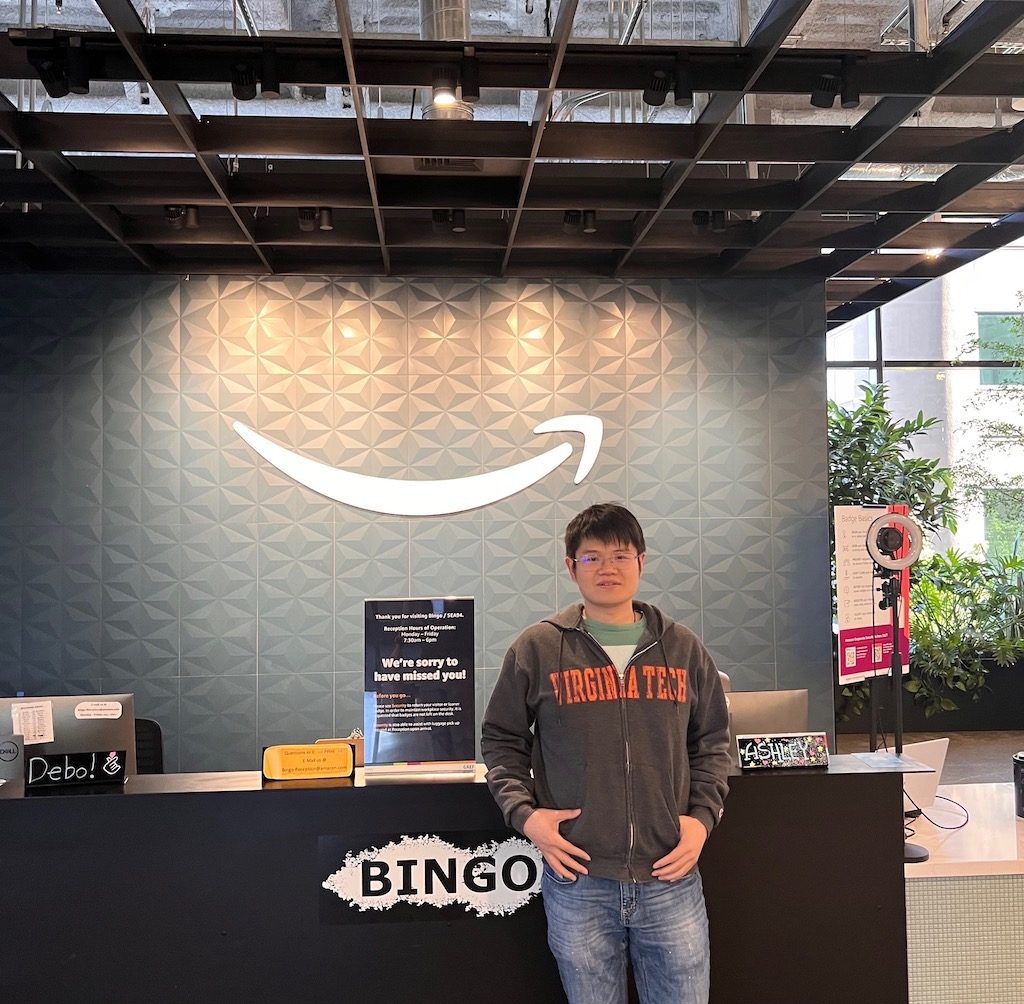
Summer offers an opportunity for graduate students at the Sanghani Center to gain real-world experience in their research focus areas by working at major companies and labs across the country. This year these include places like Amazon AWS in Seattle, Washington; JPMorgan Chase & Co in New York City; the MIT Computer Science and Artificial Intelligence Laboratory (CSAIL) in Cambridge, Massachusetts; Bosch in Pittsburgh, Pennsylvania; and the Intel Lab in Santa Clara, California.
Following is a list of Sanghani Center students – where they are and what they are doing:
Satvik Chekuri, a Ph.D. student in computer science, is a natural language processing research intern working remotely with a Deloitte Audit and Assurance Data Science team in New York City. The team’s research focuses on the intersection of knowledge graphs and Large Language Models (LLMs) in the financial domain. His advisor is Edward A. Fox.
Hongjie Chen, a Ph.D. student in computer science, is a research scientist intern at Yahoo Research in Sunnyvale, California, working remotely with the advertising team. His advisor is Hoda Eldardiry.
Humaid Desai, a master’s degree student in computer science, is a software engineer intern at Ellucian in Reston, Virginia, working remotely. He is contributing to Ellucian’s SaaS-based solutions using React.js, Node.js, and AWS cloud technologies. His advisor is Hoda Eldardiry.
Jianfeng He, a Ph.D. student in computer science, is an applied scientist intern working onsite at Amazon AWS in Seattle, Washington, where he is researching text summarization. His advisor is Chang-Tien Lu.
Adheesh Juvekar, a Ph.D. student in computer science, is an applied scientist intern working on generative artificial intelligence onsite at Amazon in Boston, Massachusetts. His advisor is Ismini Lourentzou.
Myeongseob Ko, a Ph.D. student in electrical and computer engineering, is a machine learning research intern onsite at Bosch in Pittsburgh, Pennsylvania, where he is working on a diffusion model. His advisor is Ruoxi Jia.
Shuo Lei, a Ph.D. student in computer science, is a graduate research intern onsite at Intel Labs in Santa Clara, California. She is working on developing a new few-shot learning method for multi-modal object detection to lower the effort of human annotation, training effort, and domain adaptation while meeting accuracy requirements for industrial usage. Her advisor is Chang-Tien Lu.
Wei Liu, a Ph.D. student in computer science, is a business intelligence intern at Elevance Health in Indianapolis, Indiana, working remotely with the data analysis team. Her advisor is Chris North.
Amarachi Blessing Mbakwe, a Ph.D. student in computer science, is an artificial intelligence research associate intern at JPMorgan Chase & Co in New York City, working onsite. She is conducting research on natural language processing-related problems that involve applying Large Language Models (LLMs) in finance. Her advisor is Ismini Lourentzou.
Makanjuola Ogunleye, a Ph.D student in computer science is a data scientist intern at Intuit, working onsite with the company’s AI Capital team in Mountain View, California. He is contributing to key machine learning products. His advisor is Ismini Lourentzou.
Mandar Sharma, a Ph.D. student in computer science, is a Ph.D. software engineering intern at Google AI in Kirkland, Washington, where he is working onsite on integrating state-of-the-art in natural language processing to the services provided by Google’s Cloud AI platforms. His advisor is Naren Ramakrishnan.
Ying Shen, a Ph.D. student in computer science, is a research intern onsite at Apple in New York City, where she is working on diffusion models. Her co-advisors are Lifu Huang and Ismini Lourentzou.
Afrina Tabassum, a Ph.D. student in computer science, is a research intern at Microsoft in Redmond, Washington, working onsite. She is co-advised by Hoda Eldardiry and Ismini Lourentzou.
Chiawei Tang, a master’s degree student in computer science, is a software engineer intern onsite at Juniper Network in Sunnyvale, California. His work involves creating a simulator designed to emulate the data output from wired network devices such as routers and switches. This strategic initiative facilitates system scalability testing for developers and significantly mitigates the financial impact associated with the procurement of physical hardware. His advisor is Chris Thomas.
Muntasir Wahed, a Ph.D. student in computer science, is a research intern onsite at IBM Research Almaden Lab in San Jose, California, working on the development and application of foundation models. His advisor is Ismini Lourentzou.
Zhiyang Xu, a Ph.D. student in computer science, is an applied scientist intern onsite at Amazon Alexa in Sunnyvale, California, where he is working on improving dialogue systems. His advisor is Lifu Huang.
Raquib Bin Yousuf, a Ph.D. student in computer science, is among 25 students from 19 colleges chosen to attend the Washington Post Engineering class in Washington, D.C., this summer. He is working with state of the art artificial intelligence systems to develop new technology for the Washington Post. His advisor is Naren Ramakrishnan.
Yi Zeng, a Ph.D. student in computer engineering, is a research scientist intern onsite at Meta in Menlo Park, California, working on artificial intelligence fairness, finding ways to make state of the art AI systems more robust and responsible. His advisor is Ruoxi Jia.
Jingyi Zhang, a Ph.D. student in computer science, is a graduate intern working remotely with Amgen’s Computational Biology Group within Clinical Biomarkers & Diagnostics in Thousand Oaks, California. She is taking an active role in developing a data and analytics platform as well as participating in prostate therapeutic area translational computational biology. Her advisor is Lenwood Heath.
Shuaicheng Zhang, a Ph.D. student in computer science, is a summer intern onsite at the MIT Computer Science and Artificial Intelligence Laboratory (CSAIL) in Cambridge, Massachusetts, where he is conducting research on the generative graph foundation model. His advisor is Dawei Zhou.
Xiaona Zhou, a Ph.D. student in computer science, is a University Research Association Sandia Graduate Summer Fellow at Sandia National Labs in Livermore, California. She is onsite working on anomaly detection in time series data. Her advisor is Ismini Lourentzou.