Sanghani Center Student Spotlight: Vanshaj Khattar
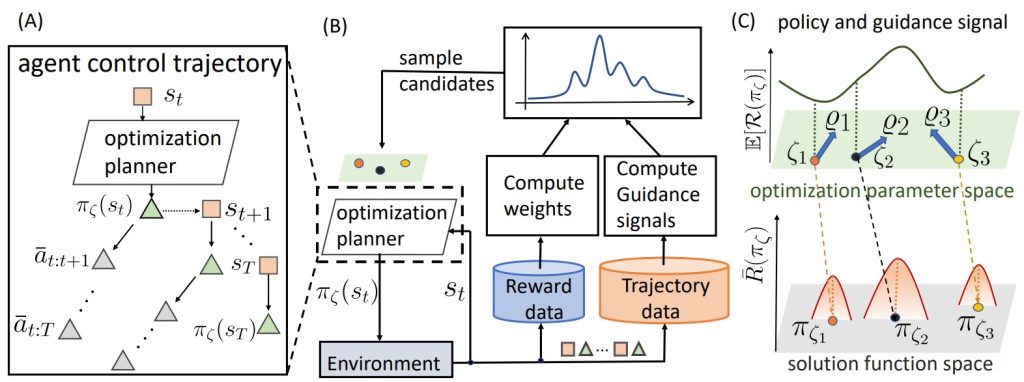
Vanshaj Khattar, a Ph.D. student in electrical engineering, is passionate about use-inspired research and solving real-world problems.
“More specifically, I am interested in how we can design trustworthy reinforcement learning algorithms that are safe, robust, explainable, and can continually adapt to non-stationarity in the real world,” said Khattar, who is advised by Ming Jin.
Currently, he is working on an offline reinforcement learning (RL) problem for building energy management, where the learning agent has to learn optimal actions from a dataset without access to the environment.
“Offline RL is hard because not all possible cases are covered inside the dataset,” Khattar said. “I am addressing this partial coverage by proposing an implicit actor-critic method for offline RL using optimization-based policies with a special robustness property to learning errors in offline RL which I am able to exploit to achieve a good performance on a multiple-building energy management problem. At the same time, I am maintaining some key aspects of interpretability which are lacking in current approaches.”
Khattar earned a bachelor’s of technology degree in electrical and electronics engineering from Delhi Technological University, India, and earned a master’s degree in electrical engineering from Virginia Tech.
While in the master’s program, he worked on motion prediction/planning for autonomous vehicles and came across reinforcement learning methods and their huge successes in many domains such as AlphaGo.
“However, I realized that the potential of RL methods was mostly being utilized in simulated domains, and real-world applications were still limited,” Khattar said. “This inspired me to pursue my research in building trustworthy RL algorithms that can be applied to real-world applications with safety guarantees.”
He said the opportunity to collaborate was a major factor in attracting him to Virginia Tech and the Sanghani Center.
In 2023, he has presented three papers: “Winning the CityLearn Challenge: Adaptive Optimization with Evolutionary Search under Trajectory-based Guidance” and “On Solution Functions of Optimization: Universal Approximation and Covering Number Bounds,” both at the 37th AAAI Conference on Artificial Intelligence; and “A CMDP-within-online framework for Meta-Safe Reinforcement Learning,” at the International Conference on Learning Representations(ICLR).
Khattar is projected to graduate in 2026 and hopes to continue his research as an industry professional.