Sanghani Center Student Spotlight: Wenjia Song
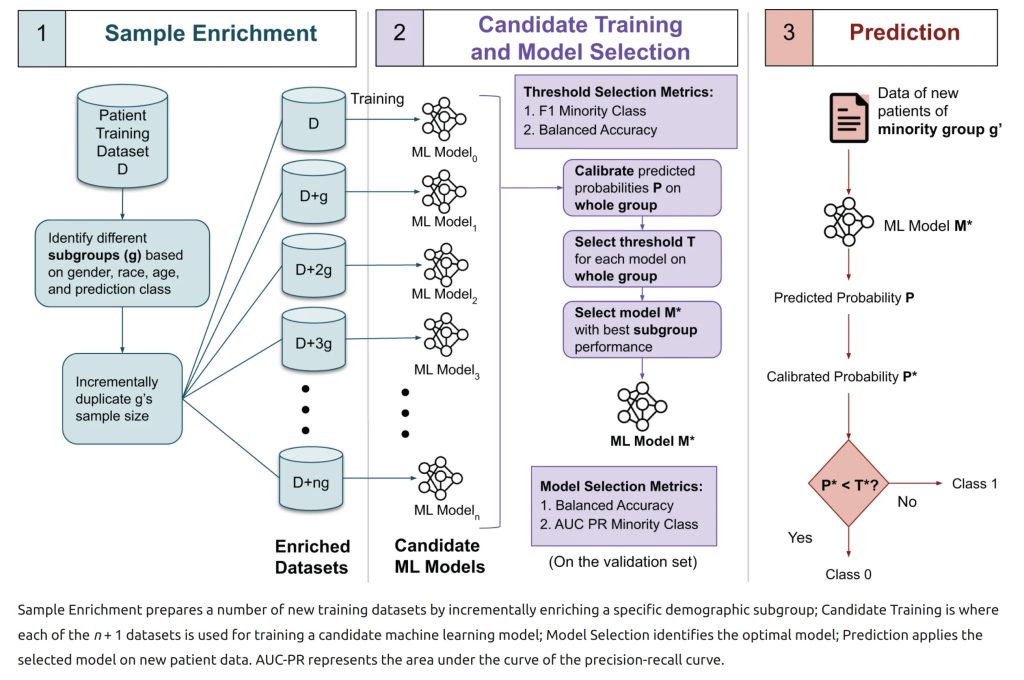
Cyberattacks have led to substantial losses for both businesses and individual users in recent years raising an urgent need to strengthen protection against such threats,” said Wenjia Song, a Ph.D. student in computer science who is working to address the problem.
“My research focuses on machine learning application and methodology development for improving accuracy on crucial detection problems, including medical predictions and threat detection in cybersecurity, through quantitative experiments,” she said.
Song’s current project is aimed at cyber threat detection.
“Real-world examples of such attacks include the Colonial Pipeline ransomware attack and the SolarWinds hack,” she said. “We try to detect malicious attack behaviors at an early stage in order to minimize the damage they may cause.”
Prior to entering the doctoral program, Song earned two bachelor of science degrees – in computer science and in mathematics — from Virginia Tech.
“As a Ph.D. student, I was attracted to the Sanghani Center because of its reputation for diverse and impactful research,” she said. “I really like being part of a thriving academic community where I receive significant encouragement and support from both professors and my peers.”
Song is advised by Danfeng (Daphne) Yao.
Among her published papers are: “Subpopulation-specific Machine Learning Prognosis for Underrepresented Patients with Double Prioritized Bias Correction,” in Communications Medicine in 2022; and “Specializing Neural Networks for Cryptographic Code Completion Applications,” in IEEE Transactions on Software Engineering in 2023.
Song also presented her work on measurement of ransomware behaviors and evaluation of defenses at both the Commonwealth Cyber Initiative (CCI)/Virginia Tech Transportation Institute (VTTI) Tech Showcase in 2022 and at the CCI Symposium in 2023.
In 2022, she presented the poster “APT Detection through Sensitive File Access Monitoring” at the Network and Distributed System Security (NDSS) Symposium and the poster “Behavioral Characterization of Crypto-Ransomware and Evaluation of Defenses” at the IEEE Secure Development Conference.
She also gave a lightning talk, “Crypto-ransomware Detection through Quantitative API-based Behavioral Profiling,” at USENIX Security 2023.
Projected to graduate in May 2024, Song said she would like to continue her research in an industry position.