Sanghani Center Student Spotlight: Raquib Bin Yousuf
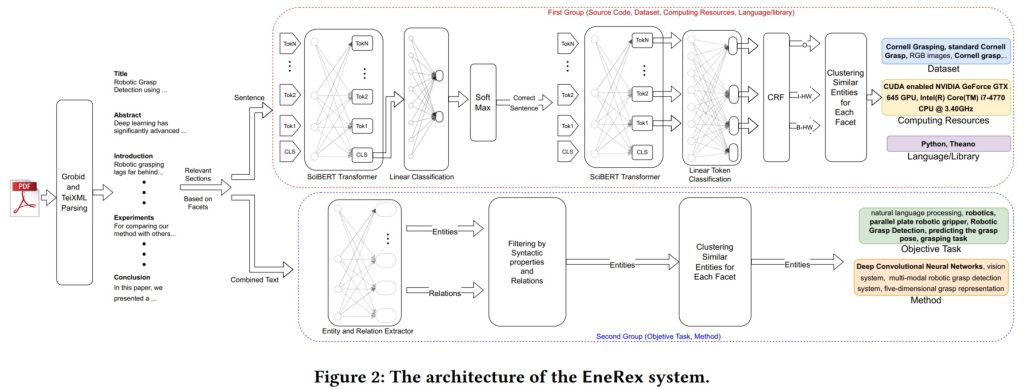
Graphic is from the paper “Lessons from Deep Learning applied to Scholarly Information Extraction: What Works, What Doesn’t, and Future Directions”
Raquib Bin Yousuf, a Ph.D. student in computer science, is exploring the capabilities of large language models to generate text from different forms of data, especially from knowledge graphs.
A knowledge graph, he said, can be a network with various entities and their relationships on any domain. Generating the correct and helpful narrative from the knowledge graphs is an important task for the user of that domain.
“Although my research focus is on natural language processing, I have been fortunate while at the Sanghani Center to work in some other multidisciplinary domains as well,” he said. “The excellent and diverse work of the faculty is what attracted me to the center and the exposure I have had to real-world problems in these collaborative projects has helped me to learn more and conduct better research.”
Yousuf’s first exposure to his research area was through information retrieval projects from large scale text data during his undergraduate years.
He has also worked on knowledge extraction projects under supervision of his advisor Naren Ramakrishnan, which have involved the application of natural language processing methods on large scale scholarly articles.
“Recently there has been a pivotal innovation in NLP in the form of the Transformer model and subsequent development of large language models,” Yousuf said. “Today’s large language models can work well, across many tasks, with little to no help at all and that has motivated me to look deep into the working nature of these state of art models for real-world applications.”
At the 2022 SIGKDD Conference on Knowledge Discovery and Data Mining last August in Washington, D.C., he presented “Lessons from Deep Learning applied to Scholarly Information Extraction: What Works, What Doesn’t, and Future Directions.” The paper explored the use of domain adapted Transformers models as building blocks to develop and deploy an automated End-to-end Research Entity Extractor, capable of extracting technical facets from full-text scholarly research articles of a large scale dataset.
Yousuf received a bachelor’s degree in computer science and engineering from Bangladesh University of Engineering and Technology (BUET) and a master’s degree in computer science from Virginia Tech.
Projected to graduate in 2025, he hopes to continue his research as an industry professional.