Sanghani Center Student Spotlight: Hongjie Chen
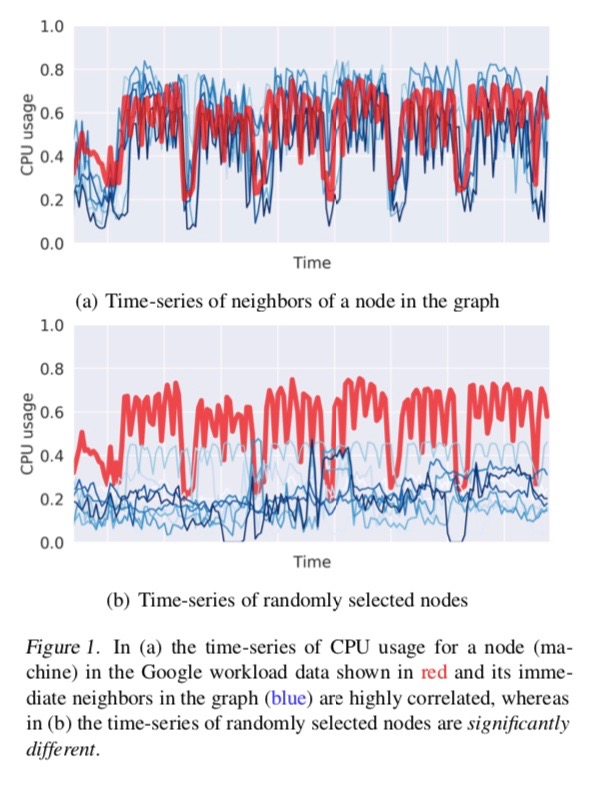
Hongjie Chen’s Ph.D. research in computer science lies in the areas of graph neural networks, time-series analysis, and recommendation systems.
“More specifically, I am currently working on time-series forecasting which is really useful in everyday life,” Chen said. “I am targeting accurate workload prediction in Cloud computing nodes.”
He said he was drawn to the Sanghani Center for its exciting advanced research atmosphere and excellent teaching faculty. He is advised by Hoda Eldardiry.
In August 2021 he presented collaborative work with researchers at Adobe Research (where he interned the summer before) and Eldardiry in proceedings of the 27th ACM SIGKDD Conference on Knowledge Discovery & Data Mining (KDD). Their paper, “Graph Deep Factors for Forecasting with Applications to Cloud Resource Allocation,” proposes a relational global model that learns complex non-linear time-series patterns globally using the structure of the graph to improve both forecasting accuracy and computational efficiency and that not only considers its individual time-series but also the time-series of nodes that are connected in the graph.
The experiments, Chen said, demonstrate the effectiveness of the proposed deep hybrid graph-based forecasting model compared to the state-of-the-art methods in terms of forecasting accuracy, runtime, and scalability,”
“Our case study reveals that GraphDF can successfully generate cloud usage forecasts and opportunistically schedule workloads to increase cloud cluster utilization by 47.5 percent on average,” he said.
Another collaborative paper, “Context Integrated Relational Spatio-Temporal Resource Forecasting,” was published at the 2021 IEEE International Conference on Big Data.
Chen earned a bachelor’s degree in computer science from Xiamen University, China. He is projected to graduate in 2024 and would like to continue working in the field of time-series analysis.