Sanghani Center Student Spotlight: Yi Zeng
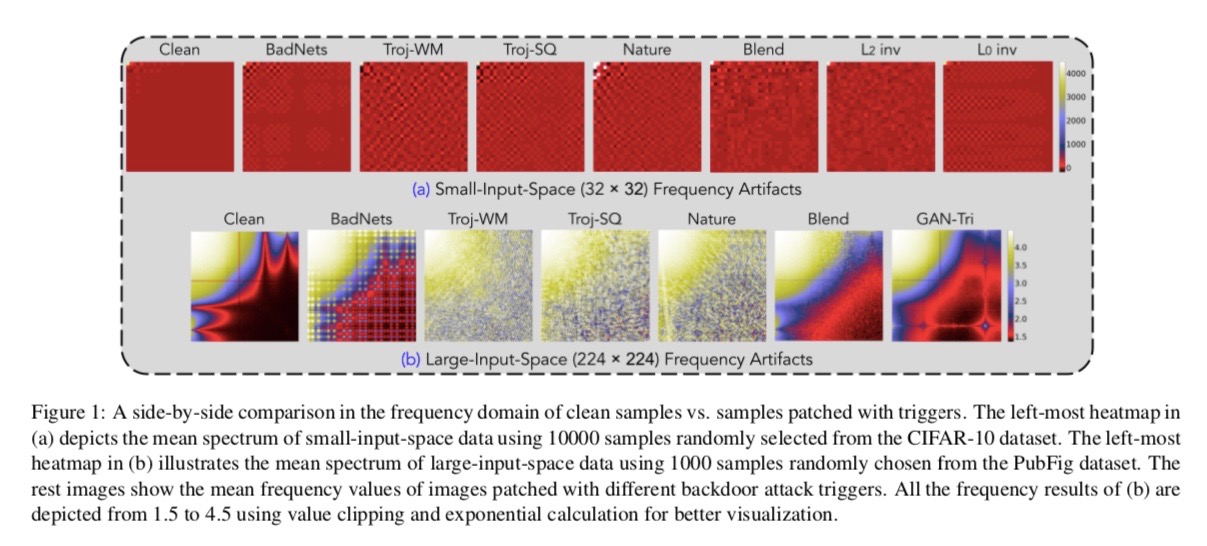
At the International Conference on Computer Vision (ICCV 2021) earlier this month, Yi Zeng, a Ph.D. student in electrical and computer engineering, gave a poster presentation on “Rethinking the Backdoor Attacks’ Triggers: A Frequency Perspective.”
Among the paper’s collaborators is his advisor Ruoxi Jia. Zeng was a master’s degree student at the University of California San Diego when he became aware of Ruoxi (at the University of California Berkeley at the time) and her achievements in trustworthy machine learning.
“When I started to look at Ph.D. programs, an internet search led me to Dr. Ruoxi’s website. By then she was at Virginia Tech and I decided to contact her,” said Zeng. “After several interactions, I knew I would like to work with her. Her unique understanding of deep learning robustness and theoretical background, along with her warm nature, are the very things that drew me to Virginia Tech and the Sanghani Center.”
Zeng said that from the beginning he has “felt the support from my department and the Sanghani Center for marketing our work on various platforms and the culture of collaboration to achieve remarkable results. I like how the Sanghani Center brings together experts in artificial intelligence and we aim for higher things to accomplish.”
Zeng’s research addresses some recent studies showing that deep learning models can be misled and evaded in ways that would have profound security implications under adversarial attacks. He is aiming to safeguard deep learning from theory to algorithm to practice and has developed several practical countermeasures that achieved state-of-the-art effectiveness with theoretical analyses.
The paper he presented at ICCV investigates backdoor attacks on deep learning models.
“Such training time attacks cause models to misbehave when exposed to inputs with specified triggers while maintaining top-tier performance on clean data,” Zeng said. “We developed a new concept and technique for unlearning potential backdoors in a model that has been backdoored. Because backdoor attacks have already demonstrated their ability to impair face recognition, autonomous driving, and authentication systems, this work can be adopted as one of the most general and optimal methods for removing and mitigating such potential security vulnerabilities in deep learning models.”
Before beginning his Ph.D. program at Virginia Tech, Zeng focused more on empirical or application efforts on deep-learning-related security issues. One intriguing aspect of empirical works revealed in recent years, he said, is that they can be easily circumvented with carefully designed adaptive attacks if the attackers know the protective pattern.
“As a result, I believe that the future of artificial intelligence security will necessitate substantial theoretical support and only by addressing the fundamental security challenges inherent in data science will we be able to unleash the full potential of data science,” he said.
Zeng presented a number of conference papers while earning his master’s degree including “Defending Adversarial Examples in Computer Vision based on Data Augmentation Techniques,” which garnered the Best Paper Award at the International Conference on Algorithms and Architectures for Parallel Processing (ICA3PP) in 2020.
Projected to graduate in 2026, Zeng would like to continue his research on the general robustness of deep learning with a more academic focus.