DAC faculty and students attend AAAI-19 conference to discuss their research
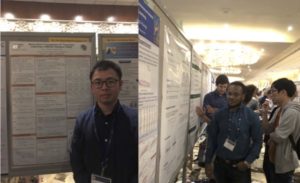
You Lu (left) and Chidubem Arachie (right), both DAC Ph.D. student in computer science, presenting their posters.
Discovery Analytics Center faculty Bert Huang and Chandan Reddy and two Ph.D. students were in Honolulu, Hawaii, last week, sharing their research with attendees at the Thirty-Third AAAI Conference on Artificial Intelligence.
Chidubem Arachie and You Lu, both in the Department of Computer Science, presented spotlight talks on studies they collaborated on with Huang, who is their advisor. The studies were also included in a poster session.
Arachie’s presentation was on Adversarial Label Learning (ALL), a method they introduced to train robust classifiers when access to labeled training data is limited. ALL trains a model without labeled data by making use of weak supervision to minimize the error rate for adversarial labels, which are subject to constraints defined by the weak supervision. Their study demonstrated that their method is robust against weak supervision signals that make dependent errors. Their experiments confirm that ALL is able to learn models that outperform the weak supervision and baseline models. ALL is also capable of directly training classifiers to mimic the weak supervision.
Lu presented Block Belief Propagation for Parameter Learning in Markov Random Fields. In this paper, the researchers developed block belief propagation learning (BBPL) for training MRF and theoretically proved that BBPL has a linear convergence rate and that it converges to the same optimum as convex BP. Their experiments show that, since BBPL has much lower iteration complexity, it converges faster than other methods that run truncated or complete inference on the full MRF each learning iteration.
Reddy gave an invited talk at the AAAI conference workshop on health intelligence, where he discussed ways to create natural language interfaces for both clinicians and patients and enable them to ask some basic questions on medical knowledge bases and clinical databases. This work, he said, will efficiently and effectively provide answers to questions and results to queries in the biomedical domain.