DAC and UrbComp students garner Deloitte Foundation Data Analytics Fellowship to fund their research
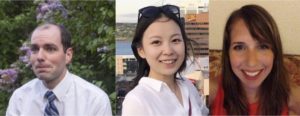
Ph.D students Jonathan Baker (left), Sirui Yao (middle) and Leanna Ireland (right).
Jonathan Baker and Sirui Yao, Ph.D. students at the Discovery Analytics Center, and Leanna Ireland, a National Science Foundation research trainee in the Urban Computing (UrbComp) Certificate program administered through DAC, have each been awarded a Deloitte Foundation Data Analytics Fellowship in the amount of $10,000 to fund their research.
Baker, also a National Science Foundation research trainee in the UrbComp program, is a math major advised by Mark Embree, professor of mathematics, associate director of the Virginia Tech Smart Infrastructure Laboratory, and DAC faculty.
Yao is a computer science major advised by Bert Huang, assistant professor of computer science and DAC faculty.
Leanna Ireland, a sociology major, is advised by James Hawdon, professor and director of the Center for Peace Studies.
The three are among five graduate students — selected from applications received from across five colleges at Virginia Tech — to receive this interdisciplinary fellowship in support of the university’s Data and Decisions Destination Area vision.
A committee consisting of four members of Data and Decisions and three representatives from Deloitte chose the fellowship recipients for 2018-2019.
Baker’s project was motivated by disasters like the 1995 collapse of a large department store in Seoul, South Korea, which killed 500 people and injured 1400. In spite of the fact that a few hours before the collapse, occupants began to feel vibrations from the air conditioning system throughout the building, no evacuation was ordered.
A building equipped with vibration sensors and software could prevent such a disaster in several ways. First, by monitoring the global vibrations of the building, software should be able to automatically detect even small amounts of structure damage so that repairs can be conducted long before evacuation becomes necessary. Second, once vibrations indicate that they building is in danger of collapse, the system could trigger an alarm, just as smoke detectors may automatically signal evacuation. Lastly, the building could use vibrations to help occupants respond intelligently to an ongoing evacuation in response to any emergency. Foot-traffic vibrations can also be used to estimate the locations of occupants and calculate real-time evacuation routes that minimize crowding, help prevent stampeding, and ensure that the building is emptied as quickly and safely as possible. The building may also be able to detect circumstances that would make some exits unavailable and adapt its evacuation directions accordingly.
By triggering the alarm and giving evacuation instructions, a smart building takes the role of emergency personnel: the building itself is the first responder. The goal of this project is to develop algorithms that this kind of intelligent building would require.
Yao’s project is focused on recommender systems.
Recommender systems play an important role in supporting human decision making. However, it is important to be aware of the potential impact of applying such technology, especially to areas that involves humans. Fairness is a crucial aspect to be taken into account. Since recommender systems are trained on data collected from the real world, which already has a long history of human bias, such data can be severely contaminated and historical biases passed on or reinforced through recommender models. It is unethical to make recommendations that constantly favor one group over the others. More concretely, unfair treatment of users would cause poor user experiences and could lead to legal trouble.
Yao’s research proposes to establish methods for measuring, analyzing, and mitigating unfairness in recommender systems. The goals are threefold: (1) to quantify and evaluate unfairness; (2) to identify the causes of unfairness; (3) to promote fairness. The success of this research will have significant impact on the wide-reaching technology of recommender systems and the many aspects of society they affect.
Ireland’s research involves crime-fighting and crime-control mobile and web applications that the general public can, for example, use to submit tips and/or share photos directly to the police.
Official crime statistics are often patchy and can be plagued by missing data, biased reporting and other measurement aliments. Crowdsourcing data can account for some of these limitations in official and self-reported crime data sources, such as lagged, incomplete, or often skewed data. However, there is also some apprehension that crowdsourced data-sources could include false-reports, trolls, and the misidentification of offenders. Relatedly, minority voices could be under-represented.
To address the potential differences in crowdsourced-policing and official policing initiatives, Ireland will investigate how the crowd-sourced initiative called the French Quarter Task Force (FQTF), colloquially known as the “Uber for cops,” impacts official crime reports. And, does success of the FQTF lead to greater community engagement, and if so, how, if at all, does the FQTF affect biased reporting? With advantages and disadvantages in both types of data, drawing from both formal and crowd-sourced data could present a clearer picture of the occurrence of crime in society, suggesting the need to include all data sources in criminological research.
The other two Virginia Tech students receiving the Deloitte Fellowship are Kaveh Kelarestaghi, a civil engineering major, and Long Xia, a business information technology major.
“A special thank you to Deloitte for initiating this interdisciplinary fellowship for our graduate students and for supporting the Data and Decisions Destination Area vision,” said Robin Russell, a member of the Data and Decisions Stakeholders Committee and chair of the Deloitte Foundation Data Analytics Fellowship Selection Committee, in a letter announcing the recipients. “We look forward to seeing the results of the research projects and engaging with these talented students.”
The Data and Decisions Destination Area seeks to advance the human condition and society with better decisions through data and to be a global destination for data analytics and decision sciences, integrating across all Destination Areas and Strategic Growth Areas of the university.